Avoiding Bias Large Random Error
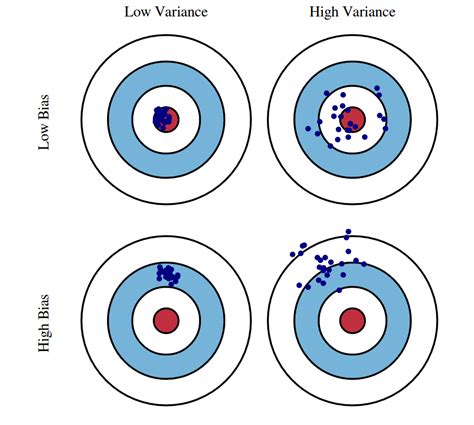

Introduction to Avoiding Bias and Large Random Error
When conducting research or analyzing data, two significant challenges that researchers and analysts face are bias and large random error. Bias refers to any systematic error introduced into sampling or testing by selecting or encouraging one outcome or answer over others. On the other hand, large random error occurs due to the natural variability in the data, which can lead to incorrect conclusions if not properly managed. In this post, we will explore strategies for avoiding bias and minimizing large random errors to ensure the reliability and validity of research findings.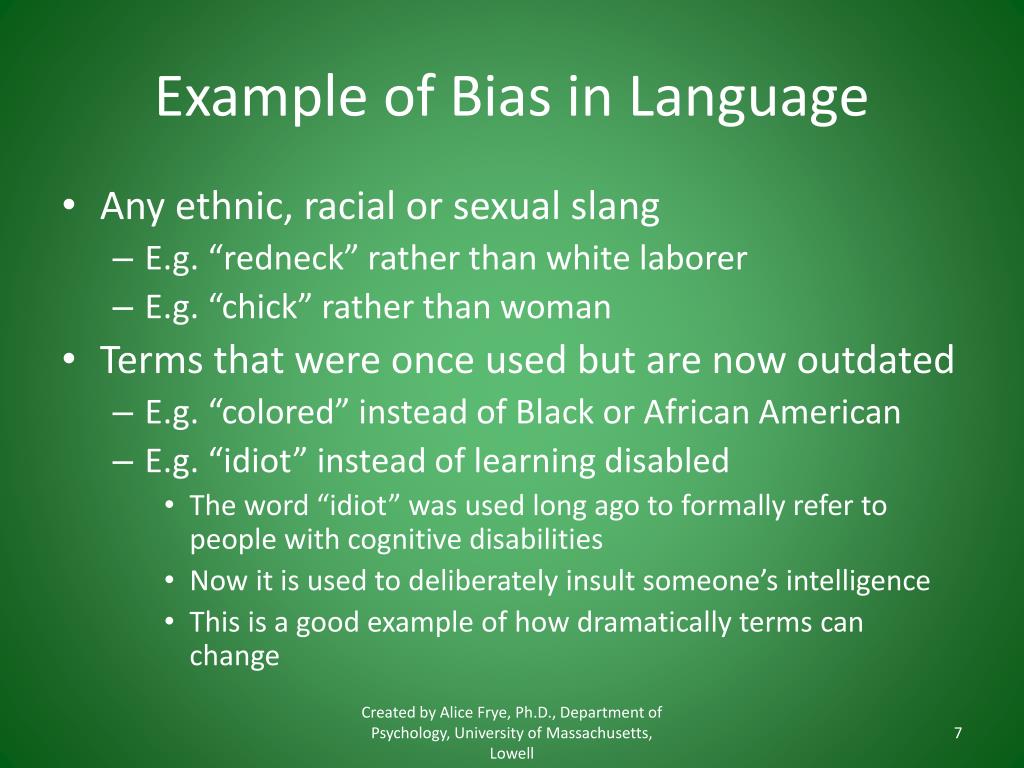
Understanding Bias
Bias can manifest in various forms throughout the research process, from the formulation of the research question to the interpretation of results. Sampling bias, for instance, occurs when the sample is collected in such a way that some members of the intended population are less likely to be included than others. Information bias arises when data collection methods introduce errors, such as recall bias in surveys where participants may inaccurately remember past events. To mitigate these biases, researchers must carefully consider their study design, ensuring that the sampling frame, data collection tools, and analytical methods are free from biases.
Strategies for Avoiding Bias
Several strategies can help in avoiding or reducing bias: - Random Sampling: Selecting participants randomly from the population can help reduce sampling bias. - Blinded Studies: Researchers can be blinded to the treatment assignments to prevent any subconscious bias in data collection or analysis. - Pilot Testing: Testing data collection tools on a small group before the main study can help identify and rectify potential biases. - Data Validation: Verifying the accuracy of the collected data against other sources or using data validation techniques can minimize information bias.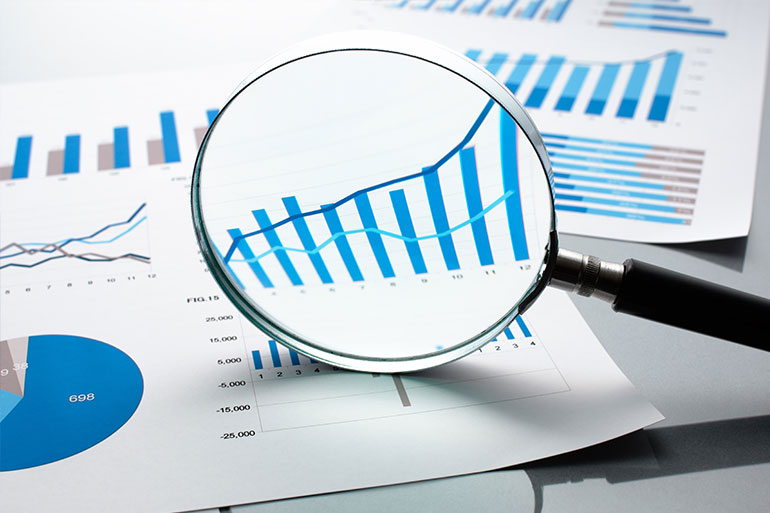
Understanding Large Random Error
Large random error refers to the variability in measurements or outcomes that occurs by chance. This type of error can lead to fluctuations in results, making it difficult to discern true effects from random variations. Sampling variability is a common source of random error, where the sample statistics (e.g., mean, proportion) differ from the population parameters due to the chance nature of sampling.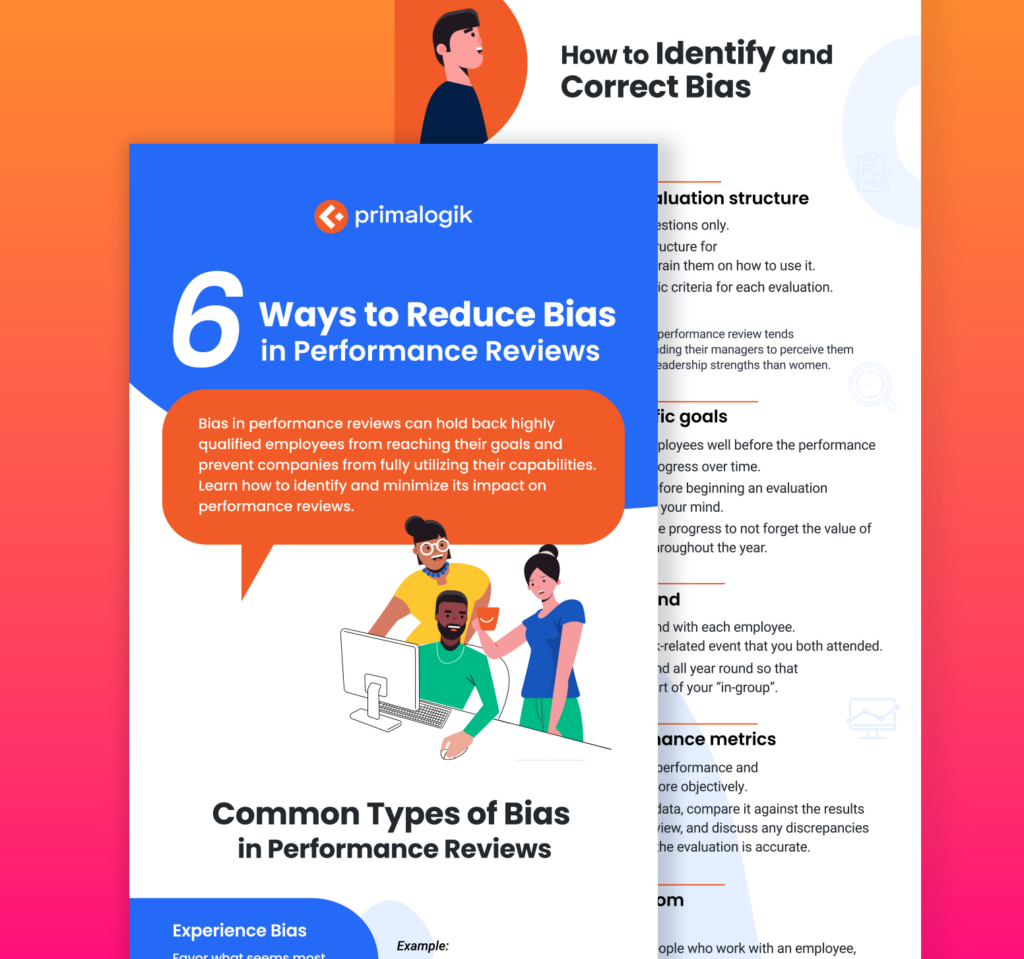
Minimizing Large Random Error
To minimize large random errors, researchers employ several tactics: - Increasing Sample Size: Larger samples tend to have less variability in their statistics, providing a more precise estimate of the population parameters. - Improving Measurement Precision: Using high-quality, reliable instruments for data collection can reduce the variability in measurements. - Replication: Repeating experiments or studies can help confirm findings and reduce the impact of random errors. - Statistical Analysis: Applying appropriate statistical techniques, such as hypothesis testing and confidence intervals, can help quantify and manage the uncertainty associated with random errors.
Best Practices for Data Analysis
In addition to avoiding bias and minimizing random error, following best practices in data analysis is crucial: - Data Cleaning: Ensuring that the data is accurate, complete, and consistent. - Exploratory Data Analysis: Initial analysis to understand the data distribution, identify outliers, and visualize relationships. - Model Validation: Testing the analytical models on separate data sets to evaluate their performance and generalizability. - Interpretation of Results: Carefully considering the findings in the context of the research question, study limitations, and potential biases.
Technological Tools and Resources
The advent of technology has provided researchers with powerful tools to manage bias and random error. Statistical software such as R and Python libraries offer advanced methods for data analysis and modeling. Data visualization tools help in identifying patterns and outliers. Moreover, collaboration platforms facilitate the sharing of methods and results, promoting transparency and peer review.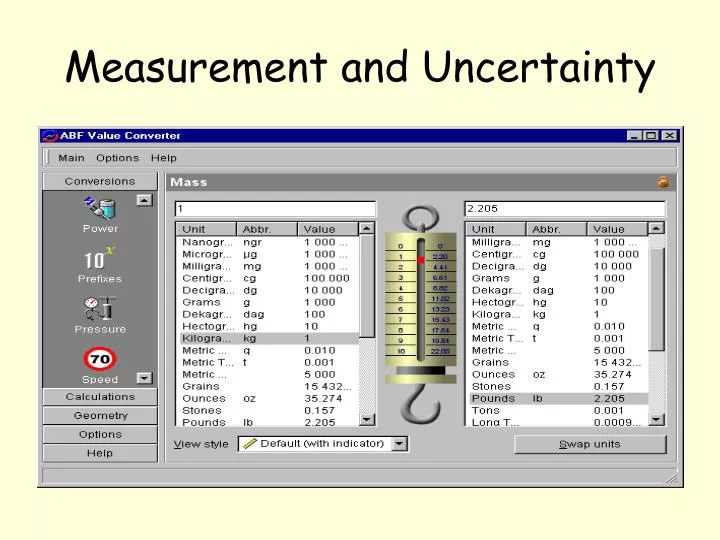
Strategy | Description | Benefits |
---|---|---|
Random Sampling | Selecting participants randomly | Reduces sampling bias |
Blinded Studies | Researchers are unaware of treatment assignments | Minimizes bias in data collection and analysis |
Increasing Sample Size | Larger samples for more precise estimates | Reduces sampling variability |
๐ Note: The choice of strategy depends on the research question, study design, and available resources. It's essential to consider the ethical implications and potential limitations of each approach.
In wrapping up the discussion on avoiding bias and large random error, itโs clear that a combination of meticulous study design, careful data analysis, and adherence to best practices can significantly enhance the validity and reliability of research findings. By understanding the sources of bias and random error and applying appropriate strategies to mitigate them, researchers can increase the confidence in their conclusions and contribute meaningfully to their fields of study.

What is the primary difference between bias and large random error?
+Bias refers to systematic errors that skew results in one direction, whereas large random error is due to natural variability in the data, leading to unpredictable fluctuations in results.

How can increasing the sample size help in research?
+Increasing the sample size reduces sampling variability, providing more precise estimates of the population parameters and enhancing the reliability of the findings.
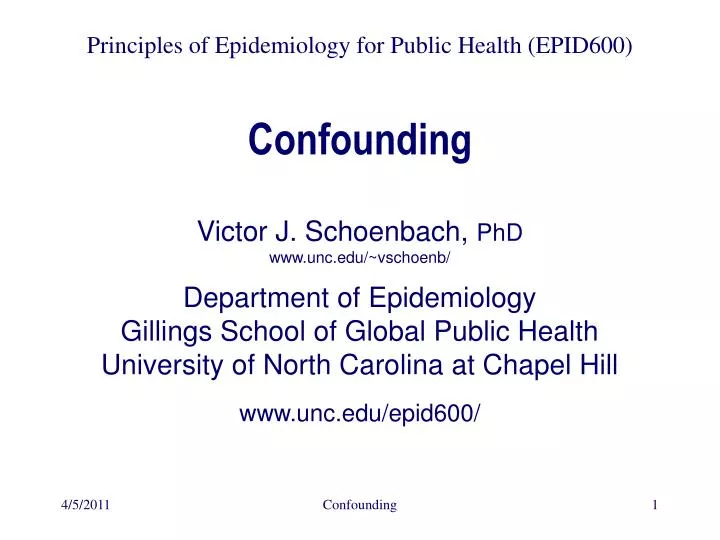
What role does data validation play in minimizing bias?
+Data validation is crucial for ensuring the accuracy and consistency of the data, thereby minimizing information bias and enhancing the overall quality of the research.